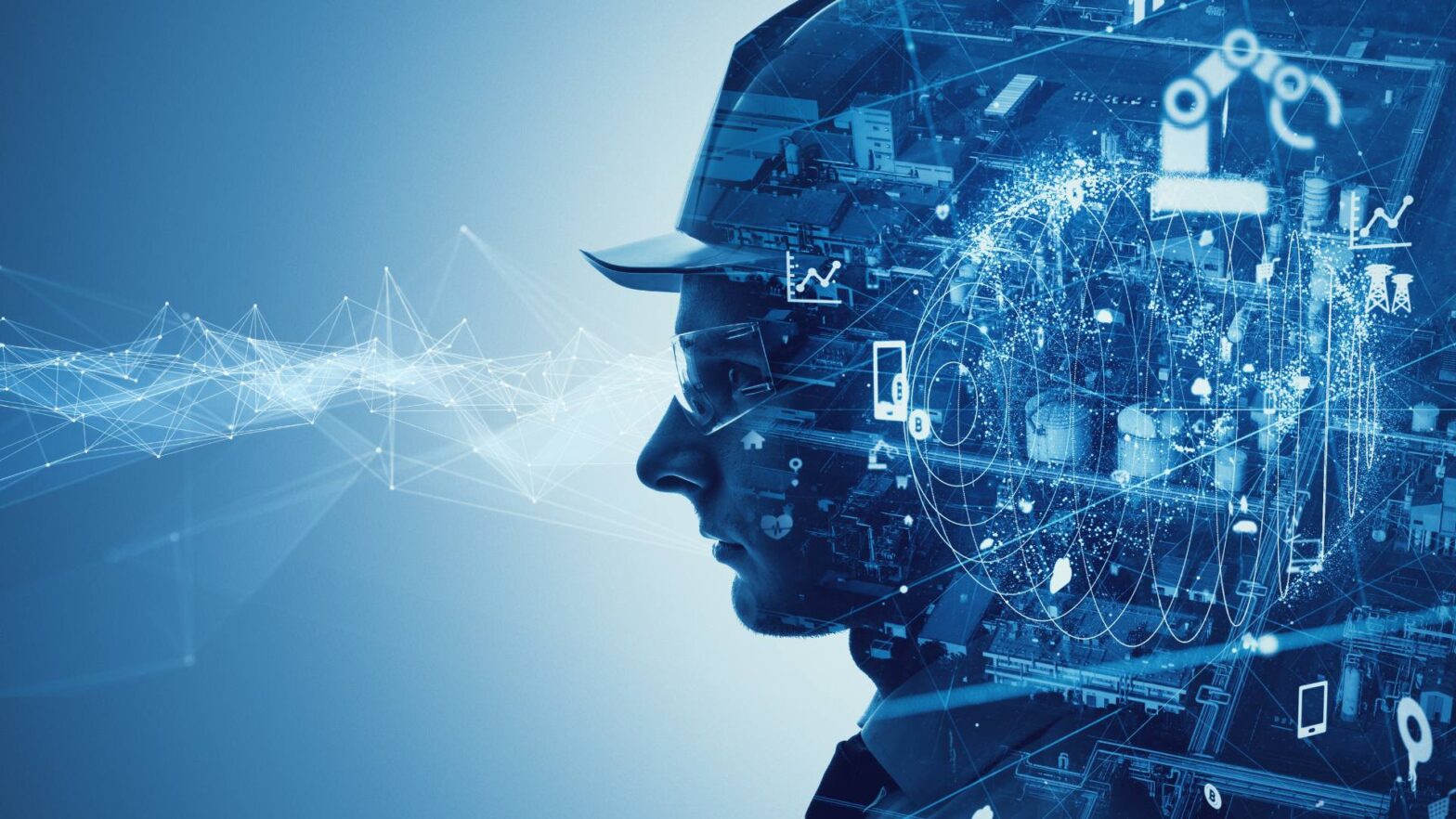
19/04/2023
Exploring the Interpretable Properties of Gated Modular Neural Networks in Industrial Data Analysis
In recent years, deep learning models have become increasingly popular in various industries, including prognostics and health management for Industry 4.0. Nevertheless, a major challenge of these models is their interpretability, which is important for domain experts or users to understand the underlying mechanisms and gain insights.
In their paper ‘Towards interpreting deep learning models for Industry 4.0 with gated mixture of experts‘, Alaaeddine Chaoub, Christophe Cerisara, Alexandre Voisin, and Benoît Iung proposed the use of the Gated Mixture of Experts (GME) to interpret a deep learning model trained on industrial data. Unlike monolithic deep learning models, GMEs actually enable the decomposition of parts of the models, potentially making them interpretable by domain experts or users. In order to test this paradigm, the AI-PROFICIENT team transformed a model that provided state-of-the-art performances on a standard industrial benchmark for predicting the remaining useful life of an asset. Alaaeddine Chaoub, Christophe Cerisara, Alexandre Voisin, and Benoît Iung validated that the transformed model’s performances were not degraded, and that the resulting model segments and clusters the data streams, according to an emerging concept that reflects previously published analyses by experts on this specific dataset.
At the same time, the AI-PROFICIENT team identified potential weaknesses of this paradigm, particularly the excessive variability of the resulting decomposition across experiments. In order to overcome this, they proposed modifying the loss function with a new knowledge-based constraint term that encodes a known prior distribution of latent concepts in the data. They found that this term enables greater control over the GME, and results in a decomposition of significantly better quality on their benchmark.