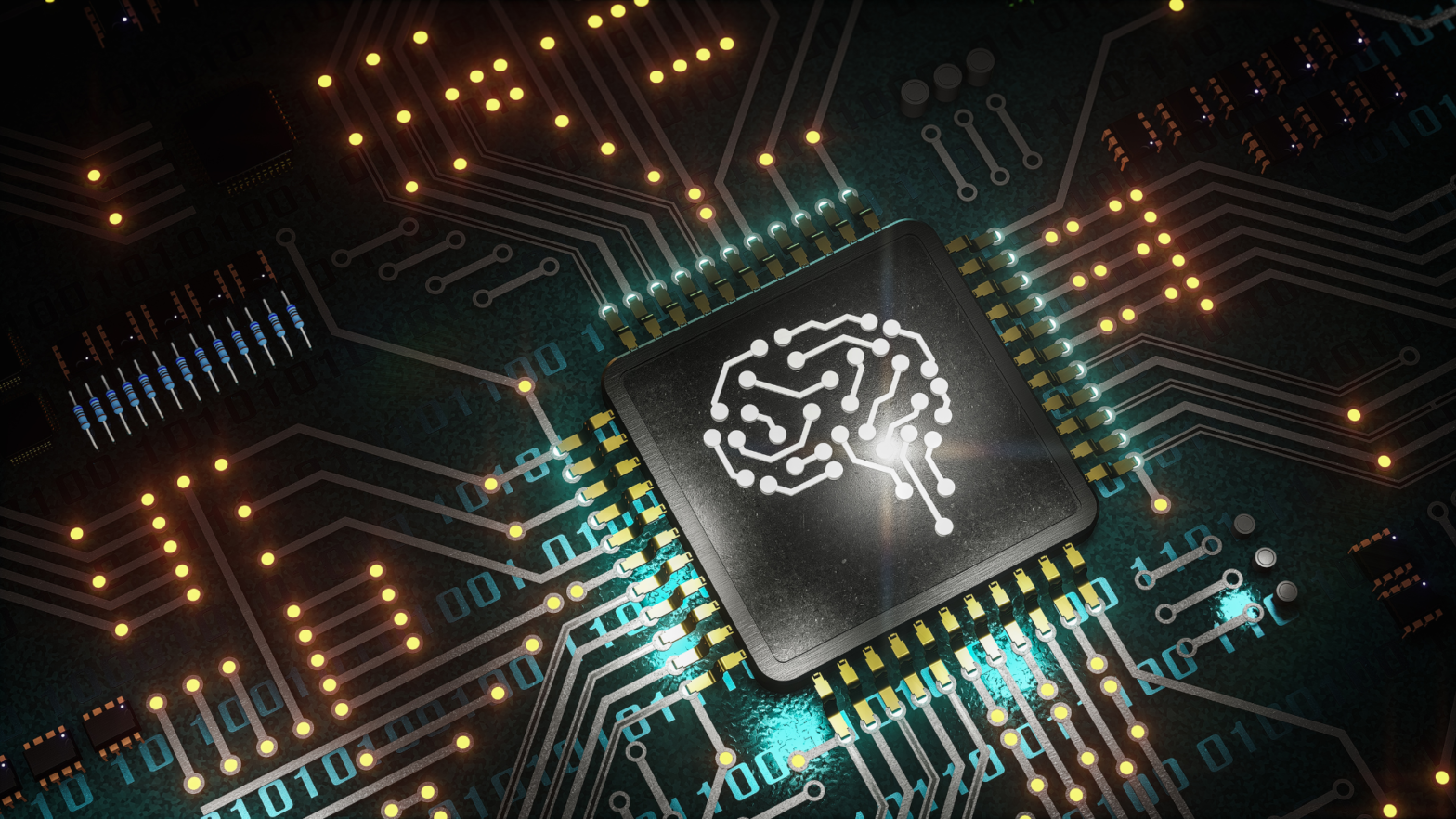
27/01/2023
Optimising maintenance policies for multi-component systems
Currently, in manufacturing, massive useful data about health condition and maintenance is often available thanks to Industry 4.0 technologies. However, taking advantage of historical data in order to optimise maintenance policies for multi-component systems has been considered as a challenge for quite some time.
As Van-Thai Nguyen, Phuc Do, Alexandre Voisin, and Benoît Iung explain in the paper ‘Artificial-intelligence-based maintenance decision-making and optimization for multi-state component systems’, this is true ‘when maintenance cost models at component level are not available, and/or maintenance actions are imperfect’. In order to cope with this issue, the AI-PROFICIENT team proposes an artificial-intelligence-based maintenance approach which first constructs a predictor based on artificial neural network (ANN) for estimating maintenance cost at system level, and then employs a customized multi-agent deep reinforcement learning algorithm to optimize maintenance decisions that can be applied for large-scale systems.
To evaluate the performance and scalability of this proposed maintenance approach, in this work, numerical studies are conducted on a small 4-component system with different configurations, and also on a large system composed of 15 components considering both deterministic and random maintenance quality.
We must point out that the simulation results show that ANN-based predictor is efficient for maintenance cost forecasting and multi-agent deep reinforcement learning is a promising solution for maintenance decision-making and optimization.